What Can AI Do For Organizations and Leaders?
- John C. Reynolds, PhD
- Oct 18, 2024
- 5 min read
This article is the second installment of a series titled "Leading in The Algorithmic Revolution: The Impact of Artificial Intelligence on Leadership"
Leaders Need To Know
AI is the topic de jour for many organizations and leaders in both the corporate and nonprofit sectors. In a daily business news update on June 26, 2023, the following statistics were reported:
Big Tech leaders mentioned “AI” 168 times in recent earnings calls.
The techy Nasdaq’s gains were above 30% this year, significantly outperforming the S&P 500.
AI-associated corporations such as Nvidia, Symbiotic, and C3.ai have soared.
1 in 4 new unicorns this year are AI startups.
The benefits for organizations leaders to evaluate this meteoric rise in awareness and utilization after almost 50 years is critical for leaders to comprehend.
A few of the more common benefits include:
Data-driven Decision Making
Algorithms are able to analyze vast amounts of data to derive insights that inform decision-making processes. They help identify patterns, trends, and correlations that decision-makers may overlook especially when considering the speed of technology against that of the human brain. With the abundance of data now available, and the growing power of algorithms to analyze and extract insights from it, leaders who utilize algorithmic tools such as AI are gaining new capabilities to make more informed and data-driven decisions. In the continuum of data, data plus interpretation is recognized as information, information plus experience or what we call knowledge, and lastly, this knowledge applied for new value or change (perhaps wisdom), algorithms are quickly becoming a leader’s choice tool for accessing fast and accurate information, to which the leader can then add their experience, aiding them in creating wise and informed decisions. Although a very good tool, algorithms are still not heuristical (or not yet), and so human insight, emotional intelligence, subjectivity, and discernment are still needed. Algorithms will inform you every time that tomatoes are a fruit (try ChatGPT); however, by experience, we know they still don’t taste very good in a fruit salad - that’s wisdom!
Personalization
Algorithms are able to develop rules and processes to customize and even personalize experiences and services based on an individual's preferences and behavior. This ability enables tailored recommendations (Netflix), targeted advertisements (Amazon), and “customized” user interfaces (your bank) to enhance user satisfaction and engagement. Zalando, a German fashion retail company, was founded as an online shoe store in 2008. Today, Zalando is Europe’s biggest wholesaler, delivering 1.4 million products to 23 countries and 49 million customers from more than 5,800 brands. Zalando combines fashion and technology by employing AI to (1) optimize logistics and fraud detection; and (2) customer-related use, such as size recommendation and personal shopping experience. Zalando employs over 100 researchers who work specifically on machine learning, with some focusing on customer needs and how to make their experience better using AI. In 2018 Zalando launched Algorithmic Fashion Companion (AFC), a machine-learning algorithm that identifies different items of clothing and makes suggestions for an outfit based on previous purchases by the customer. Zalando then “trains” AFC from the thousands of orders its customers make each day, as well as through the 200,000 outfits created specifically by stylists from the company's own personal styling service called 'Zalon'.
Optimization
Algorithms are utilized to optimize business processes, efficiently allocate resources, and maximize performance. They are currently being used to identify the most efficient solutions to complex problems, such as route optimization, inventory management, and supply chain logistics, resulting in increased efficiency and productivity. Leaders can and should, leverage these technologies to identify repetitive tasks to automate, ways to delegate routine responsibilities to machines, and certainly utilize algorithms to allocate resources more effectively. As an example Zalando uses AI to ensure that its logistical issues are solved as efficiently as possible, avoid fraud, and adjust to seasonal demand. AI is an integral part of warehouse logistics, too, including sorting, packing, and delivery. Robots at fulfillment centers have been assisting humans by identifying and picking up items and delivering them to specific packing stations, while smart algorithms have allowed for a better understanding of how and when the parcel will be delivered to the customer, depending on the type of delivery method that was chosen. Optimization of processes enables people to be displaced to roles of creativity and innovation, rather than beijing replaced. An important communication for Leaders to their workforce as AI is introduced to their organizations.
Predictive Capabilities
By analyzing historical data, algorithms are programmed to make predictions and forecasts about future events, or at minimum, the most likely outcomes in the future. AI has enhanced predictive analytics in fields like finance, healthcare, weather forecasting, and inventory demand forecasting. The most visible use of analytics to most organizational leaders is observed in marketing, however, significant predictive analytics are being used in supply chain management, risk management, fraud detection, cybersecurity, healthcare, and equipment maintenance. FedEx as an example, utilizes predictive analytics for delivery optimization and robotic delivery navigation, where machine learning capabilities enable its same-day delivery robot to detect and adjust for obstacles en route. Another case study is that of Celebal Technologies in Jaipur, India. System failure prediction of computers, workstations, servers and the network in research organizations, healthcare organizations, and banking organizations can highly benefit if they can accurately predict when their systems may fail. The adverse effects of computer failure can be mitigated to a certain extent if the proper prediction is made beforehand.
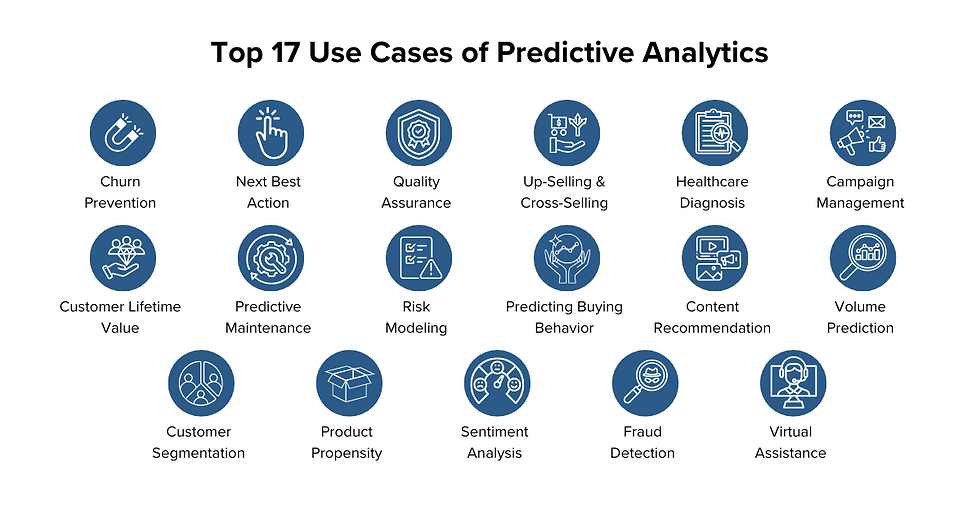
Scalability
A major value-add of algorithms is that they can easily be scaled to compute large amounts of data. Being able to efficiently process and analyze massive datasets aids the solution of complex problems at a much larger scale. An expanding customer base is a positive indicator for a growing business, but is often seasonal and demands accurate scaling. Customer service is often an issue in scaling staff to accommodate the flexible consumer base and the culture of instant gratification. For consumers, Chatbots are becoming the standard AI interface to manage, triage, and provide customer service in many organizations in real-time. Tidio (WordPress), Meena (Google), or Blenderbot (Facebook) are three examples of major organizations utilizing Chatbots for 24/7 customer service. In an increasingly demanding, impatient, and “no contact” world, Chatbots (in most cases) have led to greater customer satisfaction.
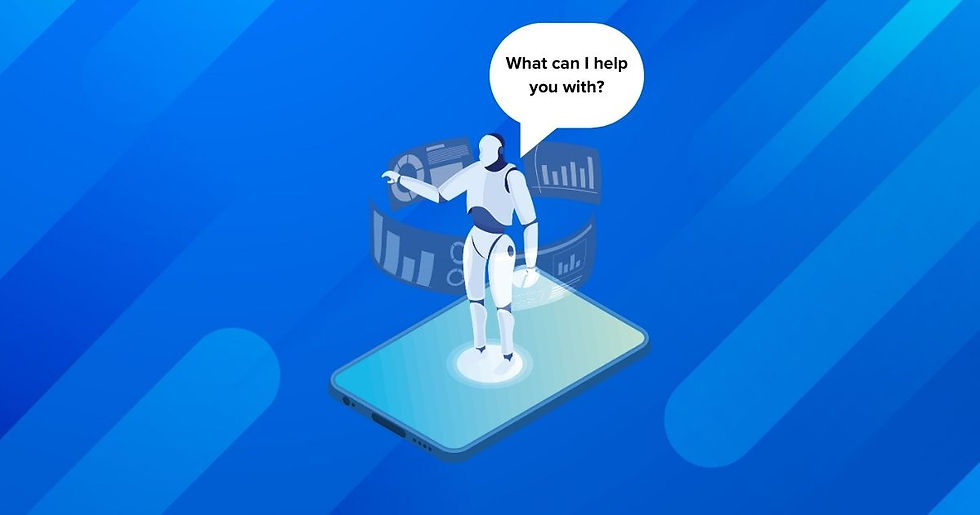
Continuous Learning
Machine learning algorithms have the ability to learn from data and improve their performance over time. AI can adapt to changing circumstances, refine its models, and make better predictions or decisions through continuous learning in a rapid and sometimes unobtrusive manner. With continuous learning AI, practical AI solutions may adapt and learn from natural drifts and variations in data environments and potentially discover better Machine Learning models. Continuous learning AI is a subset of AI solutions designed to update and improve themselves as their data, environments, and targets change. AI models interact continuously with their data environments, making them more deeply integrated in improving decisions, optimizing business processes, and understanding the characteristics of the consumer, patient, student, or worker. As an example, continuous learning is a strategy often utilized in radiology, where imaging protocols, devices, policies, and workflows change frequently. Scenarios can be developed where data, decision-making, and patient profiles vary significantly among different facilities as the algorithm “learns” more from the data and diagnosis. It is expected that continuous learning AI may predict evolving abnormalities, pathologies, and treatments that require co-evolving tools to discover new markers, diseases, and phenotypes. As a result, continuous learning AI solutions are beginning to emerge in many radiology domains, including operational, diagnostic, and predictive radiology applications.
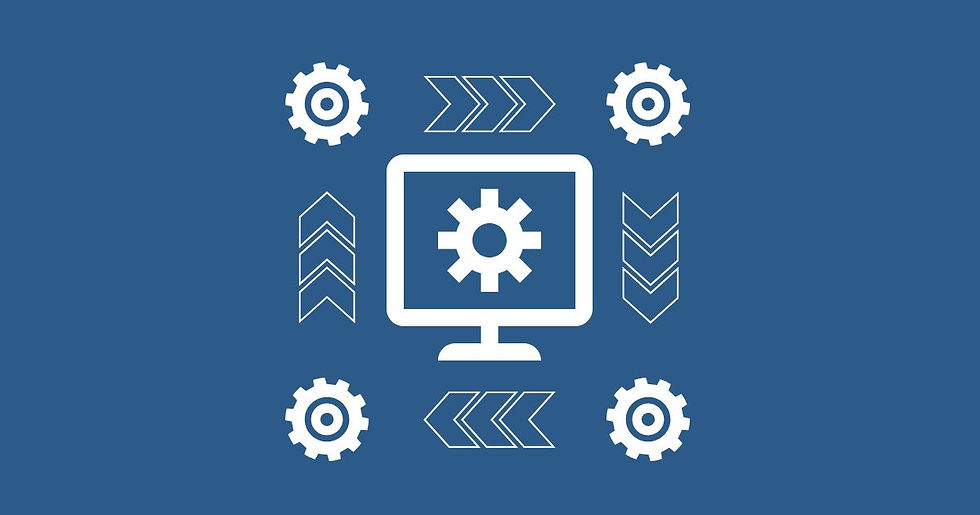
[Continued in Part 3]
コメント